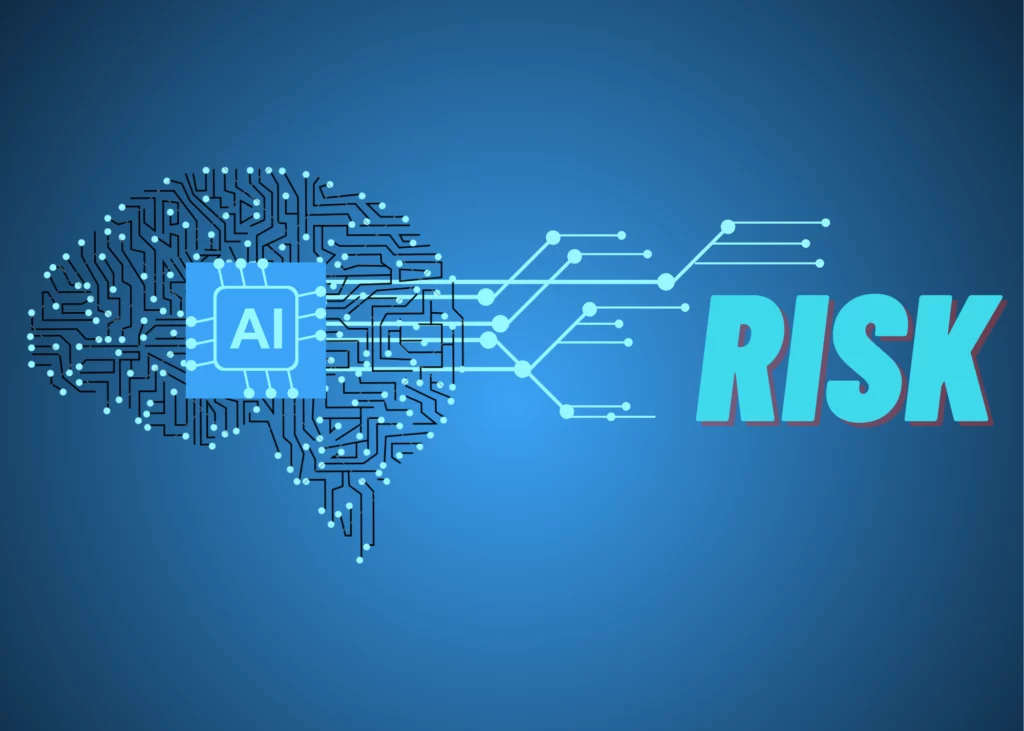
The integration of AI into healthcare is rapidly expanding, promising to transform patient care and health outcomes. Despite its potential benefits, concerns about potential negative consequences are gaining prominence as AI becomes more prevalent in the healthcare landscape. This article explores some of the risks and challenges associated with the increasing reliance on AI over humans in healthcare.
1. Injuries and Errors
A significant risk of poorly designed AI in medicine is the potential for increased medical errors. AI systems may recommend incorrect medications, overlook tumors on radiological scans, or allocate hospital beds to the wrong patients because it has wrongly predicted which patient would benefit more. These errors can result in patient injuries, and the impact of AI mistakes can be widespread compared to individual physician errors.
2. Data Availability
AI models demand substantial amounts of data for training and testing algorithms. However, healthcare data is often fragmented across multiple systems, such as electronic health records, pharmacy records, insurance claims, and consumer-generated information. This fragmentation increases the likelihood of errors, raises data collection costs, and reduces the development of comprehensive datasets all of which can limit the development of effective healthcare AI.
3. Data Privacy Concerns
AI’s reliance on vast amounts of data poses risks to patient data security and privacy. Without robust security strategies, patient data is constantly at risk. Additionally, AI has the capability to predict private patient information, even without explicit data input, raising concerns about data privacy breaches. An AI system, for instance, may identify Parkinson’s illness without any data by analyzing a person’s cursor movements.
4. Bias and Inequality
If the data used to train AI models contains any bias, it will be reflected in the AI itself. For instance, if training data is primarily from academic medical centers, the resulting AI system may be less effective for patients outside this demographic. AI algorithms may also overlook socioeconomic factors, leading to disparities in healthcare outcomes. If there is inadequate representation of physician gender or ethnicity in training data, speech recognition AI systems may not perform effectively when transcribing encounter notes. However, when patient visits are recorded by human scribes, this isn’t the case.
5. Professional Realignment
Widespread implementation of AI in healthcare, particularly in specialties like radiology, may lead to shifts in the medical profession. There are concerns that AI automation could diminish human knowledge and capacity over time, potentially making certain roles obsolete.
6. The Nirvana Fallacy
The Nirvana Fallacy, which seeks perfection rather than improvement, might be slowing down the adoption of AI in healthcare. Humans often tend to believe that a new situation or option is definitely better than the existing one, this nirvana fallacy, can be one reason that contributes to the growth of AI in healthcare. Despite the perceived benefits of AI, its potential dangers and challenges should not be overlooked.
Artificial intelligence has been the focus of much debate in recent years, despite being praised for the immense promise it holds. AI in healthcare could lead to a number of unintended and occasionally serious consequences. Moreover, addressing these challenges requires investment in infrastructure for high-quality, representative data, collaboration on FDA oversight, and modifications to medical education to prepare healthcare professionals for evolving roles. Hence, striking a balance between humans and AI is crucial for the effective delivery of care.